News Research: Automatic calculation of cervical spine parameters using deep learning
Researchers at the Schulthess Clinic have co-authored a study that examines a model that enables more accurate, automatic measurement of spinal parameters during X-rays. The model is based on deep learning and supports surgeons in particular in the diagnosis of adjacent segment disease.
Adjacent segment disease (ASD) is the development of new spinal symptoms (neurological symptoms and neck pain) due to the normal progression of degeneration (often in older patients) and/or in an area adjacent to a previous spinal operation.
Diagnosis of cervical ASD is complicated due to inaccurate correlation of X-ray images and clinical findings. Although the X-ray results may not always be consistent with the clinical diagnosis, X-ray images and clinical examination help the surgeon to decide how to treat a patient with persistent pain.
Surgeons will be assisted in making spinal assessments quicker and with a high level of accuracy
The thesis presented here takes a very technical approach and involved the use of deep learning (a form of machine learning and artificial intelligence that mimics the way in which people acquire knowledge), in order to optimise the calculation of important cervical spine x-ray measurements that our surgeons regularly perform in the clinic.
The research group developed a new analytical method using a deep learning model to accurately and automatically calculate some important spinal parameters from cervical spine x-rays. The accuracy is comparable to that of human examiners.
Conclusion: The model can help surgeons and X-ray assistants perform spine assessments faster and with a similar level of accuracy as that achieved by a human being. Although it does not completely replace the human assessor, it is a step towards improving the clinical decision-making process in the treatment of ASD.
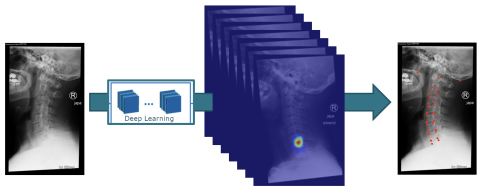
Publication: Nakarai H, Cina A, Jutzeler C, Grob A, Haschtmann D, Loibl M, Fekete TF, Kleinstück F, Wilke HJ, Tao Y and Galbusera F. Automatic calculation of cervical spine parameters using deep learning: development and validation on an external dataset. Global Spine J (Epub ahead of print) DOI: 10.1177/21925682231205352, 2023